Predicting Short-Term Rental Opportunities and Profitability with Artificial Swarm Intelligence
Advanced artificial intelligence models can accurately predict the location of future short-term rental property and maximize its profitability.
Summary
This paper presents models developed by Swarmalytics that use artificial intelligence (AI) to identify and predict the profitability of short-term rental (STR) properties. Using comprehensive data, these models have proven effective, greatly outperforming traditional methods in identifying profitable STR properties and estimating returns. The results of these models are essential for navigating the STR market, and continued improvements in the models are expected to enhance their predictive power further.
Introduction
In the rapidly evolving real estate market, the rise of STRs has introduced new opportunities in property development, management, and investment. The rise of platforms like Airbnb and VRBO has led to a surge in demand for STR properties globally. This trend has created a need for predictive models that can both identify potential STR opportunities and accurately forecast their profitability. Such models offer actionable insights for stakeholders, including builders, investors, property managers, and even policymakers.
Aiming to address these needs, this paper presents a comprehensive overview of the development and performance of two advanced analytical models designed by Swarmalytics. These models leverage AI and extensive datasets to predict STR opportunities and estimate potential profitability.
Background
The Growing Importance of STRs
The STR market has experienced substantial growth over the past decade, driven by several factors.
Technological advances: Peer-to-peer accommodation platforms like Airbnb and VRBO1 have made it easier than ever for ordinary homeowners to list their properties for rent and for travelers to find suitable accommodations2.
Travel trends: A growing preference for unique travel experiences has shifted many travelers away from traditional hotels3, increasing the demand for STRs.
Alternative investment opportunities: The STR market offers property owners and investors an alternative revenue stream compared to traditional long-term rentals. This flexibility, coupled with higher potential returns, has attracted more investors to the market4.
This growth has transformed how properties are managed and has created lucrative opportunities for property owners. However, the dynamic nature of this market requires sophisticated tools to navigate it effectively. Identifying properties with high STR potential and accurately predicting their visitor turnover are invaluable for maximizing returns on STR investment.
Challenges in STR Market Analysis
The STR market's complexity is made more challenging by various factors.
Fluctuating demand: The STR market is highly influenced by seasonal trends, with peak seasons varying by location. Special events, such as conferences, concerts, and festivals, can cause demand spikes in specific areas as well.
Regulatory changes: Cities and municipalities have introduced diverse regulations for STRs, including zoning laws, occupancy limits, and tax requirements, which can vary rapidly. Recent regulatory changes in New York City, aimed at curbing the rapid expansion of STRs to address housing shortages and community concerns, exemplify the kind of swift policy shifts that can impact the market. Similar regulatory pressures are observed in San Francisco, where local laws have been tightened to regulate the number of days a property can be rented out as an STR5.
Traditional analytical methods such as regression analyses often fall short in capturing the nuanced dynamics of the STR market. However, the vast amount of data available for analysis presents both a challenge and an opportunity, underscoring the need for advanced analytical tools. In this context, approaches like Swarmalytics' artificial swarm intelligence offer a powerful alternative to traditional methods, enabling a more effective navigation in the complexities of the STR market.
Model Development
Data Sources and Preprocessing
Swarmalytics started the model development process by gathering a comprehensive dataset encompassing properties across the United States which were converted into and listed as STRs. Specifically, properties were flagged as STR versus non-STR, and this classification was used as the dependent variable in the identification model. To create a modeling dataset for profitability estimation, this dataset was appended with profitability-related metrics such as revenue and occupancy rates. As a proxy for profitability, Swarmalytics calculated the return on investment (ROI) using STR revenue and investment amount. This metric was used as the dependent variable for the profitability model.
A massive amount of additional data related to each property’s locale was later appended to both identification and profitability estimation datasets, providing a broader array of factors for the AI to analyze. These additional data elements included business activities, geographical features, transportation metrics, climate conditions, cost of living, crime rates, health services, housing characteristics, Internal Revenue Service statistics, American Community Survey statistics, and more. The two modeling datasets were both separately split into training and validation sets, ensuring robust model development and evaluation. This comprehensive data collection and preprocessing process provided a solid foundation for model building.
Modeling Approach
Swarmalytics’ predictive models were developed using artificial swarm intelligence to train models on massive datasets encompassing various aspects of the STR market. This approach involves separating the data into homogeneous subsets, using massive parallel processing to find the best solution to the problem within each subset, and finally combining the solutions to these subsets into a comprehensive whole. These models are particularly proficient at identifying subtle patterns and interactions within the data, far beyond the reach of traditional regression analysis.
The STR identification model leverages artificial swarm intelligence techniques to generate candidate equations that compete to create accurate predictions. This model effectively scores properties on their likelihood of serving as successful STRs. Similarly, the STR profitability prediction model also uses artificial swarm intelligence to evaluate a range of features to produce a predictive output for rental yield.
Modeling Performance and Outcome
To evaluate the performance of the AI’s final models, Swarmalytics employed a variety of validation methodologies and tests. The models were evaluated based on their ability to accurately identify STR opportunities and predict profitability, with a particular focus on lift values—a metric comparing the models' predictive effectiveness against random guessing.
Properties around the country were scored by both models’ final equation, ranked from highest to lowest according to those scores, and split into 100 equal quantiles. In the top scoring quantile of the identification model, the training subset achieved a lift of over 1300, meaning that the model was 13 times more effective than random chance in pinpointing a STR property, displaying exceptional accuracy. The validation subset scored at 1200 in the top quantile, again indicating strong and reliable performance. Similarly, the STR profitability prediction model also showed a significant lift in precise estimates of potential returns, with training and validation lift values in the top quantile of roughly 170 and 150, respectively. These results indicate that the profitability model can identify STR properties that are 50-70% more profitable than the average STR. The overall results of both models underscore their practical value in guiding data-driven decision-making in the STR market.
Top Features in the STR Identification Model
In the final STR identification model, one of the primary features is the loan-to-value ratio, which shows a strong negative correlation with STR suitability, suggesting that properties with lower loan-to-value ratios are more likely to be viable STRs. Additionally, the model considers the occupancy history of the property, where properties that have been frequently occupied have a strong negative correlation with STR potential. Looking at this from another perspective, it indicates that less frequently occupied properties might offer better opportunities for STR conversion.
Top Features in the STR Profitability Prediction Model
The profitability prediction model for STRs reveals several critical factors that influence the potential profitability of STR properties. Key among these is the property's assessed value, which has a strong negative correlation with profitability, indicating that properties with higher assessed values may yield lower ROI as STRs. Access to oceans and lakes also plays a significant role, with both variables showing a strong positive correlation with profitability. Another top feature is the number of bathrooms on a property, which has a strong positive correlation with profitability, highlighting the importance of adequate facilities in attracting short-term renters.
Conclusion
Swarmalytics' models provide robust predictive capabilities for the STR market, offering valuable insights into both identification and profitability. The identification model accurately scores properties on their likelihood of serving as successful STRs, while the profitability model predicts rental yield, allowing stakeholders to make comprehensive and informeddecisions.
Moving forward, Swarmalytics aims to refine these models further, integrating additional economic factors and advanced text mining techniques to enhance predictive accuracy and adaptability. These continued developments will provide even more actionable insights for stakeholders in the STR market, contributing to informed decision-making and successful investments.
About Swarmalytics
Swarmalytics was founded in 2021 by CEO Doug Newell and CTO Dan Koehler with the goal of using artificial swarm intelligence to power insights and create value. To learn more about Swarmalytics, please visit www.swarmalytics.com.
Citations
Guttentag, D. (2015). Airbnb: disruptive innovation and the rise of an informal tourism accommodation sector. Current Issues in Tourism, 18(12), 1192-1217.
Zervas, G., Proserpio, D., & Byers, J. W. (2017). The rise of the sharing economy: Estimating the impact of Airbnb on the hotel industry. Journal of Marketing Research, 54(5), 687-705.
Oskam, J., & Boswijk, A. (2016). Airbnb: the future of networked hospitality businesses. Journal of Tourism Futures, 2(1), 22-42.
Gajdošík, T. (2019). Smart Tourism: Concepts and Insights from Central Europe.
Zaveri, M. (2023). New York City’s crackdown on Airbnb is starting. here’s what to expect., The New York Times. Available at: https://www.nytimes.com/2023/09/05/nyregion/airbnb-regulations-nyc-housing.html.
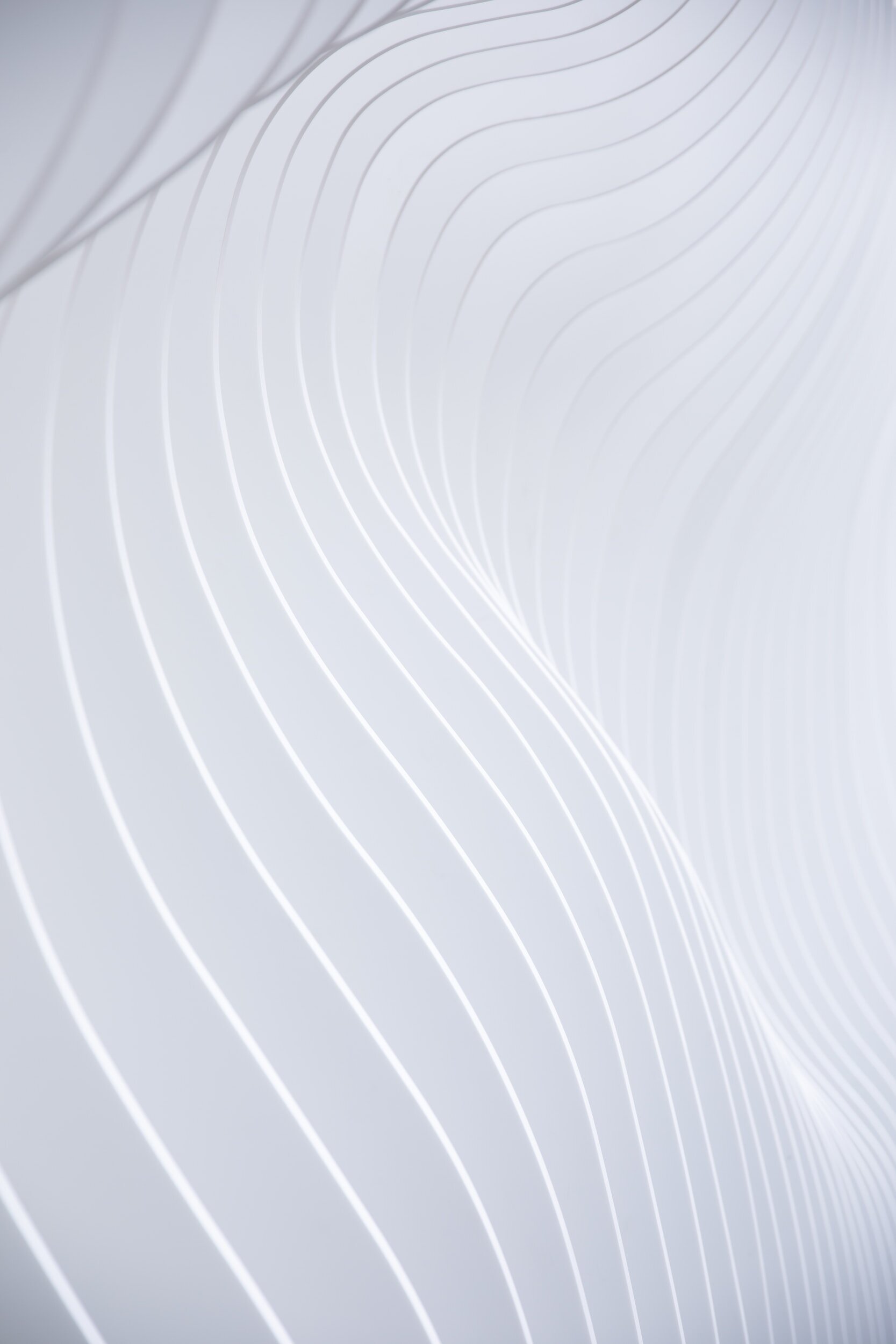