Predicting New Apartment Construction with Artificial Swarm Intelligence
Advanced artificial intelligence can predict the location of future apartment buildings around the United States.
Summary
This whitepaper presents a comprehensive overview of the development and performance of artificial swarm intelligence designed to predict the conversion of land parcels into apartments in the United States. By leveraging large datasets and approaches based in machine learning, Swarmalytics aims to enhance predictive accuracy and provide valuable insights for builders, developers, investors, and policymakers involved in the real estate market.
Introduction
In an era where the dynamics of urban development are rapidly evolving, including the shift towards remote working and the reevaluation of office space usage1, the ability to predict the conversion of land parcels into residential spaces, such as apartments, becomes increasingly significant. Swarmalytics' apartment construction prediction engine offers a data-driven approach to tackle site-specific challenges faced by builders and construction companies. By integrating data on environmental suitability, market demand, regulatory compliance, cost estimation, and neighborhood factors, this tool can help builders make informed decisions that lead to successful and effective apartment construction projects. Predicting these conversions can also offer substantial benefits to other stakeholders, including optimized strategies for investors, better planning for housing demands for urban planners, and insights into urban growth patterns for policymakers.
Background
Importance and Challenges of Selecting Apartment Location
Selecting the perfect spot for apartment construction is a multifaceted decision. It requires a thorough understanding of zoning laws and careful consideration of location-specific factors such as safety, convenience, price, building size, parking availability, and much more. These elements collectively influence the feasibility and success of the development project.2
For builders and construction companies, making such decisions involves navigating a complex set of challenges that can significantly impact the project's feasibility and profitability. These include understanding market demands, ensuring site accessibility, adhering to regulatory standards, and fostering community engagement3. Transitioning to the data aspect, the modern real estate landscape offers an extensive array of data points for analysis, from demographic trends to local amenities. This wealth of information necessitates advanced analytical tools, such as Artificial Swarm Intelligence, to distill actionable insights, highlighting the crucial need for sophisticated data processing in identifying optimal apartment locations.
Artificial Swarm Intelligence
Manual analysis of this data is out of the question; data of this magnitude can only be efficiently analyzed using artificial intelligence to search for predictive relationships at extremely high speeds. Swarmalytics’ artificial swarm intelligence engine has this capability. Research into swarm intelligence and its applications dates back to the late 1980s. Examples of early milestones include the creation of Boids, an artificial life program written by Craig Reynolds in 1986 using simple rules to create a simulated flock of birds4, and the 1992 publication of Marco Dorigo's Optimization, Learning, and Natural Algorithms, which laid the groundwork for ant colony optimization algorithms5. In the decades since, the study of swarm intelligence has continued to bear fruit. For example, Southwest Airlines' efficient open-seating policy was born out of swarm intelligence, which is also used to navigate their pilots to airport gates most efficiently6. More recently, Fortune Magazine explored the power of swarm intelligence to transform the financial world in its November 2021 article Following the Flock – The Science of Meme Stocks and Manias7, and a May 2021 article from Harvard Business Review highlighted the innovative uses of swarm intelligence across companies such as Hewlett-Packard, Unilever, and Capital One8.
Model Development
Data Sources
The development of Swarmalytics predictive models began with the collection and preprocessing of a huge set of real estate data. This data set contains every residential property in the United States that is categorized as an apartment house, garden or court apartment, high-rise apartment, multi-family dwelling, or condominium. A massive amount of additional data was appended to the dataset, providing a broader array of factors for the AI to analyze. This integration includes data on traffic accidents, business activities, climate conditions, cost of living, crime rates, health services, housing characteristics, Internal Revenue Service (IRS) statistics, job availability, schools, geographical features, transportation, American Community Survey (ACS) statistics, tree canopy coverage, consumer behavior, electrical grid information, elevation, flood zones, land use, sewer systems, architectural styles, traffic patterns, and more. Notably, data alignment was meticulously performed based on 5-digit ZIP codes or 9-digit ZIP codes where applicable, ensuring a granular and precise input for later model building process. This elaborate data collection and integration empowers the model to assess and predict apartment conversion opportunities with unprecedented detail, considering every factor that might influence such developments.
Model Approach
Swarmalytics’ predictive models were developed using artificial swarm intelligence to train models on massive data sets of newly constructed apartments across the United States. This approach involves separating the data into homogeneous subsets, using massive parallel processing to find the best solution to the problem within each subset, and finally combining the solutions to these subsets into a comprehensive whole. These models are particularly proficient at identifying subtle patterns and interactions within the data, far beyond the reach of traditional regression analysis. The solution to each problem subset is formed using machine learning, wherein candidate equations are generated and then compete against one another to create more accurate predictions. This model approach ensures a thorough understanding of the variables at play, enhancing the model’s capacity to predict land parcel conversions into apartments with unmatched accuracy.
Model Performance and Outcome
To evaluate the performance of the AI’s final model, Swarmalytics employed a variety of validation methodologies and tests.
Swarmalytics measured the AI model's performance with lift, a metric that compares the model’s predictive effectiveness against random guessing. Land parcels around the country were scored by the model’s final equation, ranked from highest to lowest according to that score, and split into ten equal groups, or deciles. In the top scoring decile, our training phase achieved a lift of 866, which means the model was 8.66 times more effective than random chance in predicting apartment conversions, showcasing exceptional accuracy for the most probable scenarios. The validation phase scored at 778, again indicating strong and reliable performance. Overall, the model demonstrates exceptional accuracy in identifying the most probable land parcel conversions into apartments while showing diminished effectiveness for less likely conversions.
Variables such as owner type, lot size, year built, census tract, land market value, investor status, and assessed land value contribute to the final equation to varying degrees. This distribution highlights the model's reliance on a mix of property characteristics, ownership details, and geographic information to predict the likelihood of land parcel conversion into apartments, showcasing a comprehensive approach to understanding real estate development trends.
To further assess the AI's model practicability, Swarmalytics also conducted real-world applicability tests, specifically targeting newly constructed apartment buildings across the US. These tests were designed to measure the model's scoring accuracy in predicting successful conversions. The results were highly encouraging, as all tests returned high scores, underscoring the model's accuracy and reliability in forecasting apartment conversion opportunities. This multifaceted evaluation approach confirms the practical value and predictive power of Swarmalytics' AI-driven model in apartment development.
Conclusion
The journey of Swarmalytics' artificial swarm intelligence in predicting land parcel conversions into apartments in the United States marks a significant advancement in the domain of real estate analytics. This whitepaper has detailed the process of developing a predictive model that harnesses vast datasets and advanced machine learning techniques to forecast urban development trends. The insights garnered from Swarmalytics’ research underscore the practical implications for stakeholders across the real estate spectrum. From facilitating informed decision-making for builders and developers to enabling policymakers to anticipate and plan for urban growth, the outcomes of this project promise to shape the future of housing. Moving forward, the continuous refinement and application of such predictive models stands as a testament to the transformative power of data-driven approaches in navigating the complexities of real estate development.
About Swarmalytics
Swarmalytics was founded in 2021 by CEO Doug Newell and CTO Dan Koehler with the goal of using artificial swarm intelligence to power insights and create value. To learn more about Swarmalytics, please visit www.swarmalytics.com.
Citations
Liu, J. (2021, November 26). The top 10 cities turning old office buildings into apartments-take a look inside. CNBC. https://www.cnbc.com/2021/11/26/the-top-10-cities-turning-old-office-buildings-into-apartments.html
Cameron, J. (2015, July 1). 5 things to consider when choosing an apartment location. Apartment Agents. https://apartmentagents.com/2015/07/01/choosing-an-apartment-location/
Understanding the risks in property development. Beech Holdings. (n.d.). https://beechholdings.co.uk/news/understanding-the-risks-in-property-development/
Reynolds, Craig W. (1987). "Flocks, herds, and schools: A distributed behavioral model". Proceedings of the 14th Annual Conference on Computer Graphics and Interactive Techniques (SIGGRAPH'87). ACM. 21 (4): 25–34.
M. Dorigo, 1992. Optimization, Learning and Natural Algorithms, PhD thesis, Politecnico di Milano, Italy.
“What Can Ants Teach Us?” CBS News, www.cbsnews.com/news/what-can-ants-teach-us/. Accessed 16 Nov. 2022.
“Planes, Trains and Ant Hills.” Science Daily, 1 Apr. 2008, web.archive.org/web/20101124132227/www.sciencedaily.com/videos/2008/0406- planes_trains_and_ant_hills.htm. Accessed 16 Nov. 2022. 5. “Why Investing Pros Spooked by Market Manias Are Studying “Econophysics.”” Fortune, fortune.com/longform/meme-stocks-stonksgamestop-econophysics-market-maniasgiorgio-parisi/.
Bonabeau, Eric, and Christopher Meyer. “Swarm Intelligence: A Whole New Way to Think about Business.” Harvard Business Review, 1 May 2001, hbr.org/2001/05/swarmintelligence-a-wholenew-way-to-think-about-business
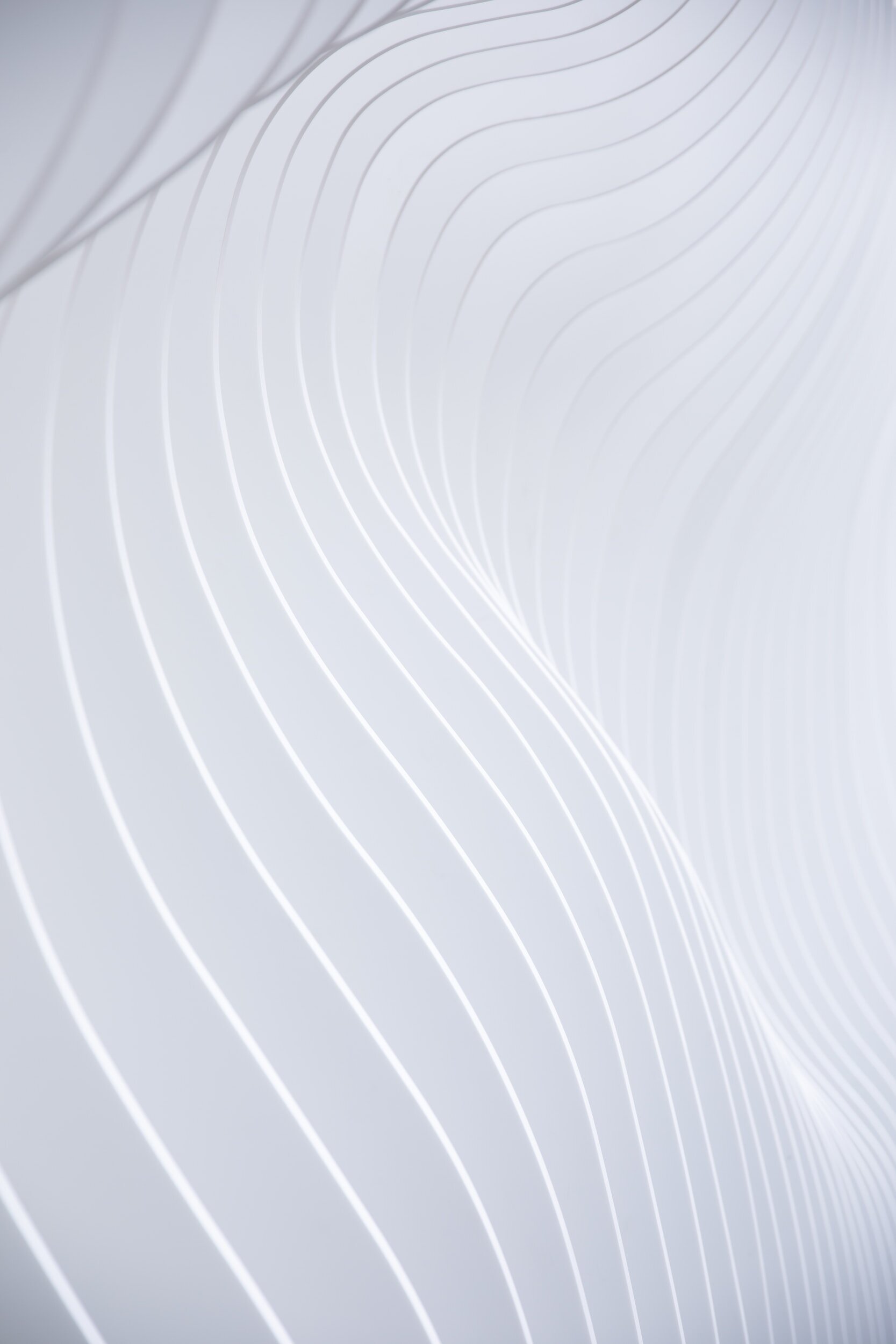